A common problem in the numerical simulation of real-world systems is the fact that exact values for the parameters of the models can exhibit a high level of uncertainty. This non-determinism in numerical models may arise as a consequence of different sources, motivating some categorization of uncertainties. Although other classifications are possible in almost the same manner, the following categorization proves to be well suited in this context:
aleatory uncertainties, such as natural variability or scatter, on the one side, and on the other side, epistemic uncertainties, which arise from an absence of information, rare data, vagueness in parameter definition, subjectivity in numerical implementation, or simplification and idealization processes employed in the modeling procedure.
All these conditions manifest as uncertain model parameters and in some situations as uncertain initial or boundary conditions. Consequently, the results that are obtained for simulations that only use one specific set of values as the most likely ones for the model parameters cannot be considered as representative of the whole spectrum of possible model configurations. Furthermore, this fake exactness provided by the numerical simulation of models with uncertain but exact-valued parameters can significantly aspect the comparison between numerical simulations and experimental testing. Namely, such a comparison may be rated as unsatisfactory if the crisp-valued simulation results do not well match the experimental ones, even though it might be absolutely satisfactory, if the uncertainties inherent to the models would have been appropriately taken into account in the simulation procedure.
While aleatory uncertainties have successfully been taken into account by the use of probability theory and, in practice, by Monte Carlo simulation, the additional modeling of epistemic uncertainties still remains a challenging topic. As a practical approach to solve this limitation, a special interdisciplinary methodology to comprehensive modeling and analysis of systems has been developed which allows for the inclusion of uncertainties – in particular of those of epistemic type – from the very beginning of the modeling procedure.
Possibility theory provides a universal framework for the analysis of systems, allowing to take the various types of uncertianty into account and to simulate them by means of fuzzy arithmetic.
Modeling
In contrast to classical probability theory, possibilistic descriptions may not only consider aleatory uncertainties, but epistemic uncertainties as well. The correct elicitation of suitable membership functions of the involved fuzzy parameters thereby forms the core of the modeling process.
Propagation
Despite modern and powerful computer structures, the prediction of system behavior under consideration of uncertainties is complex and difficult. Time-consuming and, thus, expensive model evaluations and a high problem dimension cause the simulation time to increase exponentially. However, with the help of modern, advanced methods, the computing time can be reduced by up to several orders of magnitude.
For this purpose, sparse-grid surrogate models and possibilistic multi-fidelity methods are used as approaches.
Identification
Increasing knowledge and new insights about physical systems allow more detailed and complex models describing these processes. However, the correct identification of the models' parameters is often a difficult and time-consuming task. Traditional estimation techniques rely on a structured comparison of model predictions and experimental data, whereby process and measurement noise impede an exact determination of the true values. Methods from inverse fuzzy arithmetic may capture the resulting uncertainty and quantify it accordingly.
Optimization
The optimal design of mechanical systems as well as processes for decision making under consideration of uncertainties deal with the question to what extent uncertainty and ignorance affect the optimal design. With regard to the uncertainties, a robust solution is sought.
Control
If a real system to be controlled deviates significantly from its model, poor performance or stability problems of the controller are the immediate consequence. The quantification of the uncertainty and a robust controller design may solve these problems effectively. Combining state-of-the-art control concepts and possibility theory lead to new insights and different approachtes towards robust control systems.
Software
FAMOUS (Fuzzy Arithmetical Modeling Of Uncertain Systems) is a Matlab-based toolbox for the analysis of systems with uncertain model parameters. It has been developed at the ITM and is continuously augmented with the latest results from research.
Contact
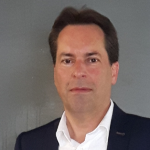
Michael Hanss
apl. Prof. Dr.-Ing.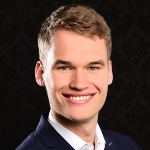