Robots are critical for progress in the automation of industry, transportation, logistics, and the service sector. Hence, to realize sustained productivity growth in industrialized societies, robots need to become better at performing their tasks and more prevalent beyond capital-intensive sectors. Our foundational research in the field of robotics contributes to the fulfillment of these two goals with multiple research projects, all of which build upon a deep understanding of the mechanics of the automation tasks and the involved systems. After all, robots are chiefly utilized to automate mechanical work. Digital twins of the robotic systems and their environment are used throughout all projects to accelerate research and development, but hardware experiments remain vital for validation.
- Cooperative Networks of Systems: A cooperating group of robots can be more robust, flexible, and versatile than a single robot or a fleet of non-cooperative robots. Cooperation is facilitated by modern communication technology, allowing the network of robots to dynamically adapt to tasks and changes. However, a robust and scalable implementation requires distributed organization and control methods without any centralized decision-making entity. We develop such methods, mainly based on distributed optimization, and test them in mechanically challenging model problems.
- Antagonistic Behavior in Networks of Systems: Using cooperating and communicating groups of robots in uncontrolled environments, i.e., outside confined and secured facilities, bears the risk that operation can be impaired by antagonistic robots that physically act antagonistically or spread misinformation in the communication network. We develop methods to recognize and deal with antagonistic behavior and validate them in experiments with real-world hardware.
- Data-Driven Modeling and Control of Robotic Systems: Performance-wise, model-based control, e.g., in the form of model predictive control, can be very hard to beat and is often surprisingly easy to tune. However, this is only the case if the models employed are of very high accuracy and of a complexity level fitting real-time needs. Since some physical phenomena, such as contact and friction, are very hard to model a priori and may even change over time, parts of highly accurate models need to be identified from real-world data. We develop methods for that and validate them in challenging control tasks with real-world robots. After all, data-driven research only fully comes to fruition when using real-world data and when operating on physical systems, including all their imperfections.
- Force Control: Equations of motion describing the dynamics of mechanical systems are of second order, and generally include effects such as inertia and gyroscopic forces. However, surprisingly, many control and decision-making algorithms in robotics operate on a first-order understanding of the underlying mechanical task, partly explaining surprisingly lackluster performance in highly dynamic situations or when sensitivity and dexterity are required. We strive to more broadly establish reliable force-level control through a deep, mechanical understanding of robots and tasks, and through designing robots, manipulators, and force-measuring devices specifically for force-control purposes.
- Optimal Control of Nonholonomic and Underactuated Systems: Even for humans, precise maneuvering of nonholonomic systems is challenging. Lateral millimeter-level accuracy when parking a car, let alone with a trailer, is hard to achieve quickly. Precise maneuvering of non-holonomic robots may, however, be useful to automate, e.g., high-precision measurement tasks. The situation is similar for underactuated but holonomic systems such as many kinds of boats. We research model predictive controllers tailored to such systems and validate them with real-world hardware, where we make use of an understanding of the mechanics and the geometry of the underlying task. To that end, we establish insightful links to the mathematical field of sub-Riemannian geometry.
- Data- and Response-Surface-Driven Design Assistant for Controlled Flexible Multibody Systems (related project from SPP2353): Nowadays, robots and mechanisms are often designed with many tasks in mind, and it is rare that foundationally new designs are employed. From generation to generation or to adapt to specific tasks, often only minor changes are made, sometimes even only in software. Today, this is often prudent since designing a completely new mechanism tailored to a very specific task would require months or years of highly-skilled expert work to deal well with just one specific task. However, a highly task-specific mechanism design may be much better suited to the task. For instance, it may be at the same time more accurate, cheaper to produce, and more efficient, e.g., by requiring less energy during operation or less material for production. Thus, to make it worthwhile to use mechanisms customized to specific tasks, we develop artificially intelligent design assistant systems that automate the design process. Together with available and emerging technologies in the field of additive manufacturing, this will in the future realize the vision of receiving a task-specific and manufacturing-ready mechanism design with minimal manual expert work.
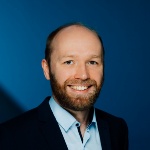
Henrik Ebel
Dr.-Ing.(2016 - 2024)
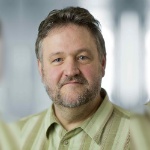
Peter Eberhard
Prof. Dr.-Ing. Prof. E.h.- Profile page
- +49 711 685 66388
- Write e-mail
- Pfaffenwaldring 9, 70569 D-Stuttgart