Robotic swarm applications generally assume that the robot agents are functional and cooperative. However, agents can be affected by hardware failures, such as malfunctioning wheels, or software errors,
Additionally, there are several security concerns in the application of robot swarms, including the identification of intruding agents, the assurance of swarm mobility and the adherence to energy constraints. Thus, detecting anomalous behavior in the physical motion of an agent can increase the probabilityDeployment task
Deployment tasks are characterized by a swarm of robots deploying in an area of variable size, for example, in the case of using a swarm for the surveillance of an area. The robots are instructed to distribute in the deployment area in such a way that the area is optimally monitored by the swarm. To ensure the optimal coverage, the robots communicate their position to the other swarm agents. However, the swarm's goal of detecting illicit activities or entities within part of the area can be prevented by strategically positioning an antagonist agent to assume control of a certain area of interest. To accomplish this goal, the antagonist can easily exploit the robot swarm’s endeavour to evenly distribute within the coverage area by moving toward the area of interest and thereby driving the other agents away from it.
Antagonistic robot types
Conspicuous deviations from normal behavior, such as a robot ceasing to move due to a hardware failure, might lead to the straightforward identification of anomalous agents. Thus, we consider different types of antagonistic agents that aim to reach their target while trying to conceal their antagonistic behavior.
The trained network can subsequently be applied to assess the robot's actions for every time step. In the deployment task, an agent that has been categorized as anomalous can be excluded from the swarm, while the remaining swarm agents adapt their positions to cover the entire deployment area.
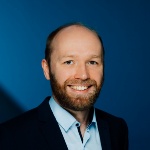
Henrik Ebel
Dr.-Ing.(2016 - 2024)
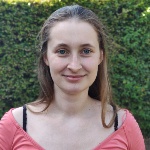