Project Description
Nowadays, hearing diagnostics are very limited in their specificity for some pathologies and in their quantitative assessment due to the large interindividual variances and the poor visual accessibility of the ear. Frequently, only a yes-or-no decision is possible, which in many cases depends to a large extent on the subjective assessment of the ENT doctor.
By evaluating objectively obtainable, non-invasive audiometric measurements with the aid of a numerical middle ear model, the hidden middle ear properties can be made visible and quantifiable. Using the model, cause-effect relationships of pathological changes on the transmission behavior of the middle ear can be investigated in a targeted manner, independent of secondary influences. Together with clinical observations, this improves physical understanding and allows phenomena to be directly related to the mechanical properties of natural structures. Furthermore, the model can quantitatively and in a multimodal manner link expert knowledge of physical relations with clinical expert knowledge. As a result, the information content of large amounts of data from various objective diagnostic procedures such as wideband tympanometry, which currently can only be evaluated to a limited extent, can be much better evaluated and linked.
Our current research focuses on a valid modeling of diagnostic measurements such as wideband tympanometry. Besides published clinical studies, temporal bone measurements are used for model validation. Temporal bone measurements enable a systematic and controlled investigation of structural changes in the ear that are difficult to detect in patients and could only be detected in long-term studies. In addition, further important measures are available, which are not measurable in patients.
In order to fit the simulation results to individual measurement data and to identify the model parameters corresponding to the anatomical properties, various identification methods such as pattern search algorithms are applied. Due to the large number of model parameters, the identification procedures are preceded by sensitivity analyses.
Methods such as Monte Carlo and Bayes inference are used to identify uncertainties and parameter distributions of different normal and pathological populations. Neural networks are used to develop practitioner-oriented diagnostic algorithms that take into account the extensive model knowledge.
Benjamin Sackmann
M.Sc.(2017 - 2025)
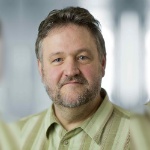
Peter Eberhard
Prof. Dr.-Ing. Prof. E.h.- Profile page
- +49 711 685 66388
- Write e-mail
- Pfaffenwaldring 9, 70569 D-Stuttgart